- AI
- 15 min read
- December 2024
How Businesses Can Harness AI for Business Intelligence
A distributor struggled with fragmented data—sales reports, inventory levels, and customer feedback scattered across systems. By the time insights were compiled, trends had shifted, and costs had risen. Manufacturers faced supply chain delays, while retailers struggled to meet growing customer demands across online and offline channels. These challenges lead to inefficiencies, rising costs, and missed opportunities.
The solution? AI-powered Business Intelligence (BI). AI transforms fragmented data into actionable insights through automation, predictive analytics, and real-time monitoring. No wonder 80% of companies are investing in AI to improve efficiency and decision-making. For example, a retailer reduced inventory waste by 20%, saving millions annually with predictive analytics.
“The ultimate aim of AI is not just automation but amplification—helping leaders make smarter, faster decisions.” – Satya Nadella
AI-powered BI bridges the gap between challenges and opportunities. Predictive analytics reduces inventory waste by 20%, real-time dashboards cut stockouts by 40%, and AI-driven insights boost repeat purchases by 25%, driving growth and loyalty.
Fragmented data no longer needs to hold you back. Start by identifying bottlenecks in your current processes and explore scalable AI for Business Intelligence solutions. Transform challenges into strengths and lead your business into the future with smarter decisions, streamlined operations, and sustainable growth.
AI in Business Intelligence: Key Technologies and Impact
1. Understanding AI's Role in Business Intelligence
AI enhances Business Intelligence (BI) by analyzing large volumes of data to uncover patterns, trends, and insights. While BI focuses on transforming raw data into actionable information, AI takes it further by automating data analysis, providing predictive insights, and improving decision-making. This integration empowers organizations to make smarter, faster, and more strategic decisions, driving growth and efficiency.
2. Key Components of AI in Business Intelligence
Machine Learning
Machine learning allows systems to learn from historical data, predicting market trends and customer behaviors to optimize operational strategies.
Natural Language Processing (NLP)
NLP enables machines to interpret and process human language, facilitating the analysis of unstructured data such as customer feedback and improving engagement strategies.
Predictive Analytics
Utilizing historical data and AI algorithms, predictive analytics forecasts outcomes, enhances risk assessment, and enables proactive business decisions that drive competitive advantage.
Computer Vision
This technology interprets visual data, helping businesses analyze patterns such as customer traffic, optimize layouts, and improve overall operations to drive sales and efficiency.
Deep Learning
Deep learning leverages neural networks for processing large datasets, uncovering insights that inform high-level strategic decisions.
3. How AI Transforms Data into Insights
AI is transforming the way businesses unlock value from their data, empowering leaders like you to turn vast and complex information into clear, actionable insights. By analyzing structured, unstructured, and real-time data, AI uncovers patterns, predicts trends, and delivers insights, enabling faster decisions and greater efficiency while creating a competitive edge.
To truly grasp this transformation, explore the process shown in the image below—your roadmap to smarter AI-driven decisions.
I. Data Collection and Cleansing: AI-first gathers data from various sources like CRM systems, social media, IoT devices, and sales platforms. It then cleanses the data, removing inconsistencies and irrelevant information ensuring that only high-quality, usable data is analyzed.
II. Pattern Recognition and Predictive Analytics: Once the data is prepared, AI identifies patterns and correlations that might not be visible to the human eye. Using machine learning models, it can recognize trends from historical data and predict future outcomes—whether it's customer behavior, market fluctuations, or supply chain needs.
III. Real-time Decision Support: AI-powered tools then provide real-time insights based on live data streams, allowing leaders to make immediate, data-driven decisions. For example, AI might identify a sudden drop in customer satisfaction or a spike in demand, enabling decision-makers to adjust strategies on the fly.
IV. Actionable Insights: Ultimately, AI doesn't just present data—it transforms it into actionable insights that drive strategy. These insights might help refine marketing strategies, optimize pricing, improve customer segmentation, or streamline operations. AI makes it easier for business leaders to identify opportunities, minimize risks, and act on data-driven intelligence.
For business leaders, this capability means AI isn't just a tool for analysis; it's an enabler of strategic decisions that move the needle. By leveraging AI for business intelligence, companies can automate complex analysis, reduce human error, and focus on turning insights into sustained competitive advantage.
Industry-Specific Applications of AI in Business Intelligence
AI applications turn business data into actionable insights, optimizing operations, driving smarter decisions, and enhancing competitiveness across industries.
1. AI in Retail Business Intelligence
AI-driven BI solutions are transforming retail by enhancing customer experiences, streamlining inventory management, and improving sales forecasting and marketing. Retailers are becoming more agile and data-driven through machine learning and AI technologies.
I. Customer Segmentation & Personalized Marketing
AI is revolutionizing how retailers understand and interact with their customers. Machine learning algorithms can analyze customer behavior, demographics, and purchasing patterns to create detailed customer segments. Retailers can use this information to tailor marketing campaigns and product offerings, enhancing personalization and increasing conversion rates.
- Case Study: AI-powered recommendation engines, like those used by Amazon and Netflix, integrate AI for business intelligence to analyze customer data—such as past purchases and browsing history—enabling personalized product suggestions that boost sales, enhance satisfaction, and foster repeat business.
II. Inventory Management and Demand Forecasting
AI-enhanced business intelligence (BI) tools revolutionize inventory management by leveraging AI-based demand forecasting to predict demand at a granular level. These tools enable businesses to ensure optimal stock levels, avoiding overstocking and stockouts. By analyzing historical sales data, seasonality, and external factors such as weather patterns or market events, machine learning models provide precise demand predictions. This capability transforms inventory management into a strategic advantage, showcasing how AI for BI drives smarter decision-making and operational efficiency in dynamic business environments.
- Case Study: Walmart uses AI to predict regional demand for products, enabling more efficient stock management. By predicting when products will be in high demand, the retailer can adjust inventory levels proactively, minimizing stockouts and reducing excess inventory.
III. Sales Forecasting
AI-driven BI tools are empowering retailers to forecast sales and improve operational planning more accurately. Machine learning algorithms take into account various factors, such as previous sales trends, market conditions, and consumer behavior, to predict future sales with higher accuracy.
- Case Study: Macy's has implemented AI for business intelligence, using machine learning to enhance its sales forecasting and inventory management. By leveraging AI-driven algorithms, Macy's analyzes data from various sources, including historical sales, customer preferences, and social media trends. This enables the company to predict demand more accurately, adjust inventory levels in real time, and avoid stockouts or overstocking. As a result, Macy's can streamline operations, reduce waste, and better align product offerings with customer demand. Additionally, AI empowers Macy's to fine-tune pricing and marketing strategies, boosting sales and improving overall customer satisfaction.
AI in Manufacturing Business Intelligence
AI is transforming manufacturing with real-time insights that boost efficiency, reduce downtime, and enhance quality control, enabling faster, smarter decision-making for greater operational success.
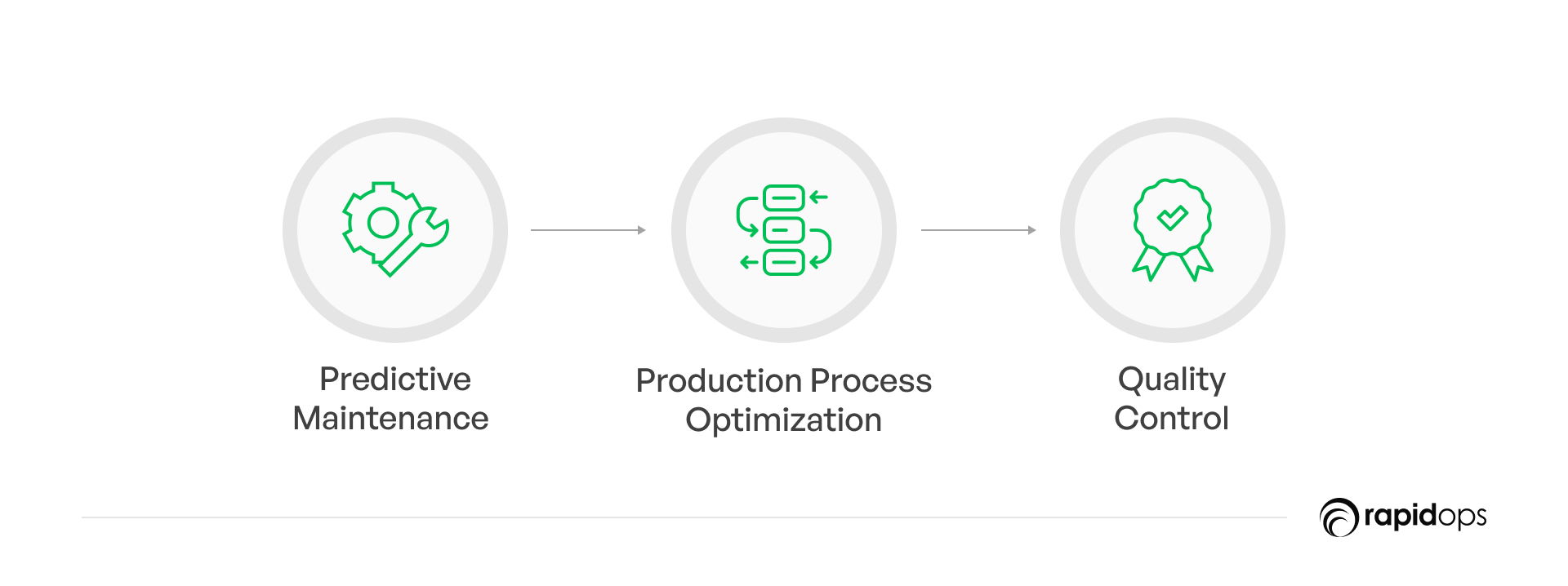
I. Predictive Maintenance
One of the most impactful AI applications in manufacturing BI is predictive maintenance. AI algorithms analyze sensor data from machines and equipment to predict when maintenance is needed, preventing unplanned downtime and costly repairs. By integrating AI into BI systems, manufacturers can schedule maintenance more efficiently, reducing disruptions to production.
- Case Study: Siemens MindSphere uses AI to collect and analyze real-time data from industrial machines, predicting potential failures and alerting maintenance teams ahead of time. This predictive approach minimizes the risk of machine breakdowns, optimizes production and reduces costs associated with unexpected downtime.
II. Production Process Optimization
AI in BI systems also allows manufacturers to optimize production workflows. By analyzing real-time data from production lines, AI algorithms identify inefficiencies and recommend adjustments to improve throughput, reduce waste, and streamline operations.
- Case Study: Automotive manufacturers like Ford use AI-powered BI systems to monitor production lines in real time, identifying potential bottlenecks and optimizing workflows. These systems enable manufacturers to improve production speed while maintaining high-quality standards.
III. Quality Control
AI is also enhancing quality control processes in manufacturing. Machine learning algorithms can analyze vast amounts of production data to detect anomalies and variations that may indicate defects, ensuring that only high-quality products reach the market.
- Case Study: General Electric uses AI-based visual inspection systems to monitor products during the manufacturing process. These systems can detect defects that may not be visible to the human eye, ensuring higher quality control standards and reducing the number of faulty products.
AI in Logistics and Distribution Business Intelligence
AI is revolutionizing logistics by optimizing route planning, inventory tracking, and distribution management. This boosts operational efficiency, reduces costs, ensures timely deliveries, and improves customer satisfaction, enabling businesses to leverage AI for business intelligence and make smarter, data-driven decisions.
I. Route Optimization
AI-driven BI tools enable real-time optimization of delivery routes, saving fuel and time while improving delivery accuracy. Machine learning models analyze various data sources—such as traffic, weather, and road conditions—to provide the most efficient delivery routes, ensuring quicker and cost-effective deliveries.
- Case Studies: FedEx and UPS use AI-powered route optimization tools to improve delivery schedules. By analyzing real-time traffic and weather data, these companies can adjust routes on the fly, reducing delays and ensuring that packages arrive on time.
II. Logistics And Distribution Management and Demand Prediction
AI in logistics BI systems plays a critical role in managing distribution by predicting demand fluctuations and potential disruptions. By analyzing historical data and external factors, AI algorithms can predict demand spikes, helping companies adjust their distribution strategies in advance.
- Case Studies: Amazon uses AI-powered BI systems to predict demand for products, allowing the company to adjust its inventory levels and shipping schedules. This ensures that Amazon's warehouses are stocked with the right products at the right time, minimizing stockouts and improving customer satisfaction.
III. Warehouse Automation and Inventory Tracking
AI is reshaping warehouse operations by automating key processes such as sorting, picking, and packing. By combining AI with real-time Business Intelligence (BI) analytics, businesses can enhance operational efficiency, reduce errors, and optimize inventory management. This integration empowers decision-makers to make data-driven choices that streamline operations, improve stock accuracy, and boost delivery speeds.
- Case Studies: Zara utilizes AI-powered robots in its warehouses for sorting, picking, and packing, integrated with real-time BI analytics. This automation has reduced inventory errors by 30%, increased warehouse efficiency by 40%, and improved delivery speed by 20%, enhancing overall operational performance and customer satisfaction.
Key Benefits of Integrating AI in Business Intelligence
AI and BI are two sides of the same coin—together, they can redefine how your business makes decisions, optimizes processes, and scales. Discover the game-changing benefits this integration can bring to your organization.
- Enhanced Decision-Making and Speed
Integrating AI into BI enhances decision-making by processing vast amounts of data in real time. Unlike traditional methods that may take hours or days to analyze, AI can sift through structured and unstructured data in seconds, providing instant insights that drive more informed, quicker decisions. - Predictive Analytics and Forecasting
AI for business intelligence analyzes historical data to uncover patterns and trends, enabling businesses to predict future behaviors and market shifts. This insight empowers organizations to make proactive decisions, optimize strategies, and plan with precision for long-term success. - Improved Data Integration and Quality
AI helps streamline data integration by automating the process of aggregating and cleaning data from multiple sources. This ensures businesses have access to accurate, high-quality data for their BI systems, improving the quality of insights and business decisions. - Personalized Customer Experience
AI allows businesses to gain deeper insights into individual customer preferences, enabling personalized marketing and product recommendations. This leads to higher customer engagement and satisfaction, improving overall retention rates.
Strategies for Successful AI Implementation in Business Intelligence
AI in business intelligence enhances decision-making, speeding up analysis by 5x and boosting profits by 20%. The visual outlines strategies, with actionable insights focusing on improving data quality, setting clear goals, integrating AI with existing systems, and ensuring continuous monitoring and training to maximize growth and efficiency.
1. Identify Business Needs and Objectives
The first step in successfully implementing AI in BI is understanding your business's specific needs. What problem are you trying to solve, or what opportunity do you want to seize? For example, do you want to enhance decision-making, streamline operations, or improve customer experiences? At this stage, you decide which AI use cases will best address your challenges or opportunities. By aligning AI-driven Business Intelligence with your strategic objectives, you ensure that AI delivers real, measurable value.
Actionable Insight: Clearly define measurable business objectives, such as reducing operational costs, improving sales forecasting accuracy, or increasing customer satisfaction. These objectives will guide the selection and implementation of the right AI solutions.
2. Collaborate with Experienced AI Partners
Implementing AI can be a complex and transformative process, which is why it's crucial to partner with experienced AI solution providers who understand your industry’s unique needs. Collaborating with the right vendors or consultants ensures seamless integration, effective adoption, and the realization of AI’s full potential for your business. To help you navigate this journey and make the right choice, explore Top AI Development Companies You Can Partner With (2025) for trusted partners who can drive meaningful results and accelerate your AI transformation.
Actionable Insight: Collaborate with AI solution providers who specialize in your industry, as they can offer tailored solutions and insights that maximize your chances of success.
3. Assess Data Availability and Quality
The foundation of AI for Business Intelligence is high-quality, relevant data. Assess your current data infrastructure and ensure that you have access to clean, structured, and consistent data. With quality data, AI models will perform effectively, leading to accurate insights and better decision-making.
Actionable Insight: Evaluate whether your existing data is sufficient for AI models and identify any gaps. Cleanse and integrate your data where necessary, and consider whether you need additional data sources to fill these gaps. A well-prepared data foundation is critical for AI to thrive.
4. Select the Right AI Model
After identifying business needs and ensuring data readiness, the next step is to choose the appropriate AI model. AI in BI offers a variety of tools, from predictive analytics to machine learning and natural language processing (NLP). The model you choose should align with your business goals and data capabilities.
Actionable Insight: If your goal is to predict future trends or forecast sales, predictive analytics or machine learning models would be best. If you need to analyze unstructured data like customer reviews or social media posts, NLP for sentiment analysis tools might be a better fit.
5. Ensure Scalability and Flexibility
Is your AI strategy ready to grow with your business? As data volumes increase, market dynamics shift and business needs evolve, many companies struggle to scale AI effectively. This often results in inefficiencies, slower decision-making, and missed growth opportunities. A successful strategy requires systems that handle growing complexities, integrate seamlessly with new technologies, and adapt to evolving goals, ensuring long-term efficiency and innovation.
Actionable Insights: Implement cloud-based platforms to scale effortlessly with your business growth, avoiding costly replacements. Opt for modular architectures that allow seamless expansion without disrupting operations. Ensure integration with existing and future tools to maintain flexibility and efficiency. Prioritizing these strategies enables AI to drive smarter decisions, foster innovation, and ensure long-term success in a dynamic market.
6. Implement AI in Phases
AI implementation should be timely. Start small by testing AI solutions in a pilot phase. This allows you to measure initial results, troubleshoot any issues, and refine your processes before scaling. Once the pilot shows positive results, you can gradually expand the AI applications throughout your organization.
Actionable Insight: Begin with a manageable project that addresses a specific business need. After validating the AI model's effectiveness, expand its application to other departments or use cases.
7. Focus on Data Governance and Privacy
Ensuring robust data governance is critical when implementing AI in BI. Make sure your data is secure, compliant with regulations, and free from bias. Prioritize data privacy and security to maintain customer trust while mitigating risks associated with data misuse.
Actionable Insight: Set up a comprehensive data governance framework that includes data security, privacy policies, and regular audits. Implement AI models in a way that complies with GDPR or other relevant regulations.
8. Monitor, Evaluate, and Iterate
Once your AI systems are live, continuous monitoring and evaluation are essential. AI models are dynamic and need regular updates to stay relevant as new data emerges or business goals shift. Implement feedback loops to measure AI for business intelligence performance and make necessary adjustments to enhance results.
Actionable Insight: Track AI performance using KPIs such as increased revenue, reduced operational costs, or improved customer retention. Use these insights to refine AI models and optimize them over time.
9. Measure Success and Communicate Impact
Once your AI systems are implemented, it's important to measure their impact on your business goals. Use KPIs to evaluate the performance of your AI in BI solutions and clearly communicate these results to stakeholders to demonstrate the value of your AI investments.
Actionable Insight: Present measurable outcomes such as increased revenue, cost savings, or efficiency gains to show the effectiveness of AI in BI. This will help secure buy-in for future AI projects.
Successfully integrating AI in Business Intelligence transforms data into actionable insights. By aligning AI with business goals, ensuring data quality, and selecting the right models, businesses can unlock value, drive growth, and achieve long-term success.
Overcoming Challenges in AI Adoption
Businesses that overcome AI challenges, such as data quality and integration, see up to 25% higher profitability. We’ve outlined these challenges to help you understand where your specific hurdles lie, positioning your business for long-term success and growth.
1. Selecting Between Off-the-Shelf Solutions and Tailor-Made AI Solutions
One of the first hurdles businesses face when adopting AI for Business Intelligence is choosing between off-the-shelf solutions and custom-built, tailored models. Off-the-shelf AI solutions are typically quicker to deploy and cost-effective for standard tasks. However, they may need to address specific business requirements, leading to functionality limitations fully. In contrast, tailored AI models offer greater flexibility, alignment with business goals, and customization. However, they come with higher upfront costs and longer development times.
2. Data Quality and Preparation
AI-driven BI relies heavily on clean, accurate, and well-organized data. Poor-quality data, such as inaccuracies, inconsistencies, or missing information, can lead to incorrect predictions and poor decision-making. Properly preparing and structuring data is essential to achieving reliable and actionable results from AI, ensuring that insights are both accurate and valuable.
3. Integration with Existing BI Systems
Integrating AI with any business system—whether legacy or modern—is a critical challenge. AI requires data from various systems across the organization, including CRM, ERP, HR, finance, and distribution platforms. Ensuring seamless data flow between these diverse systems is essential for AI to deliver accurate insights. Without proper integration, data silos can create inefficiencies, delays, and increased costs. Overcoming these hurdles allows businesses to unlock AI's full potential and make faster, data-driven decisions across all departments.
4. Skill Gaps and Talent Shortage
Implementing AI requires specialized knowledge in areas like machine learning, data science, and AI model development. Many businesses need help finding skilled talent internally, leading to project delays. This talent shortage often forces companies to rely on external consultants, which increases costs and extends the timeline for full AI integration.
5. Cost and ROI Concerns
The financial investment in AI can be substantial, covering expenses such as software, infrastructure, and specialized talent acquisition. Calculating ROI from AI adoption is complex, as the tangible benefits may take months or even years to manifest. This delayed return on investment can make it challenging for decision-makers to justify the upfront costs.
6. Data Privacy and Security
AI systems handle large volumes of sensitive data, raising significant concerns about privacy and security. Organizations must comply with privacy regulations like GDPR to avoid data breaches and legal penalties. Ensuring robust data protection measures is crucial to maintaining customer trust and meeting legal requirements.
7. Finding the Right Technology Partner
Selecting the right technology partner is essential for successful AI integration. Not all AI vendors are equipped to provide end-to-end solutions that meet specific business needs.
Organizations should prioritize selecting partners with proven industry expertise, advanced technological capabilities, and a track record of delivering scalable and flexible AI solutions. The right technology partner ensures seamless integration, accelerates implementation, and supports effective scaling of AI systems, driving innovation and long-term success.
Future Trends in AI and Business Intelligence
As business intelligence (BI) evolves, artificial intelligence (AI) is driving this transformation. For business leaders, understanding the potential of AI for business intelligence is essential to staying competitive. We explore the key trends shaping AI in BI, offering practical insights to help organizations harness these innovations for growth, smarter decision-making, and long-term success.
1. Generative AI: Revolutionizing Content Creation
- What It Is: Generative AI utilizes sophisticated algorithms to produce new content, such as text, images, and videos, based on extensive data input.
- Why It Matters: This technology empowers businesses to automate the creation of reports, marketing materials, and personalized communications, saving time and enhancing creativity. For example, by using generative AI for report generation, teams can focus more on strategic analysis rather than tedious data compilation.
2. Advanced Predictive Analytics: Anticipating Future Trends
- What It Is: Predictive analytics employs machine learning to analyze historical data and forecast future trends, enabling organizations to make informed decisions.
- Why It Matters: With the ability to anticipate customer behaviors and market shifts, companies can proactively adjust their strategies, optimize inventory, and personalize offerings. For instance, retailers leveraging predictive analytics can better manage stock levels, ensuring they meet consumer demand while minimizing excess inventory costs.
3. Decentralized AI for Privacy and Security
- What It Is: AI will leverage decentralized technologies like blockchain to process data in a way that ensures privacy and user control without relying on centralized systems.
- Why It Matters: As privacy concerns continue to rise, decentralized AI offers businesses a secure way to analyze sensitive data while maintaining full transparency. This innovation will enable data-driven insights without compromising user confidentiality, building trust and compliance with privacy regulations.
Your Vision, Our Expertise: Let's Create Impact Together!
Integrating AI into your Business Intelligence (BI) strategy is a vital cornerstone for achieving lasting success. At RapidOps, we understand that every business is unique, and the challenges you face require tailored solutions that truly work for you.
With over 15 years of experience, we specialize in transforming complex data into actionable insights that drive growth, optimization, and confident decision-making. We're not just a technology provider; we're a trusted partner committed to your success. From retail to manufacturing and distribution, we've helped many business leaders leverage data to make smarter decisions, streamline operations, and achieve lasting results.
Let us help you unlock the true potential of your data. Reach out for a free consultation, and together, we'll design a strategy to maximize your business's growth and success.
What’s Inside
- AI in Business Intelligence: Key Technologies and Impact
- Industry-Specific Applications of AI in Business Intelligence
- Key Benefits of Integrating AI in Business Intelligence
- Strategies for Successful AI Implementation in Business Intelligence
- Overcoming Challenges in AI Adoption
- Future Trends in AI and Business Intelligence
- Your Vision, Our Expertise: Let's Create Impact Together!